Data governance is not an IT strategy. It is a business strategy—an essential enterprise wide initiative that enables faster decisions and improves data quality. In the Public Sector, data governance must bring together key stakeholders across business and IT to link to enterprise outcomes and priorities. In this post, we’ll explore why data governance is important and explain how to implement it effectively in the public sector.
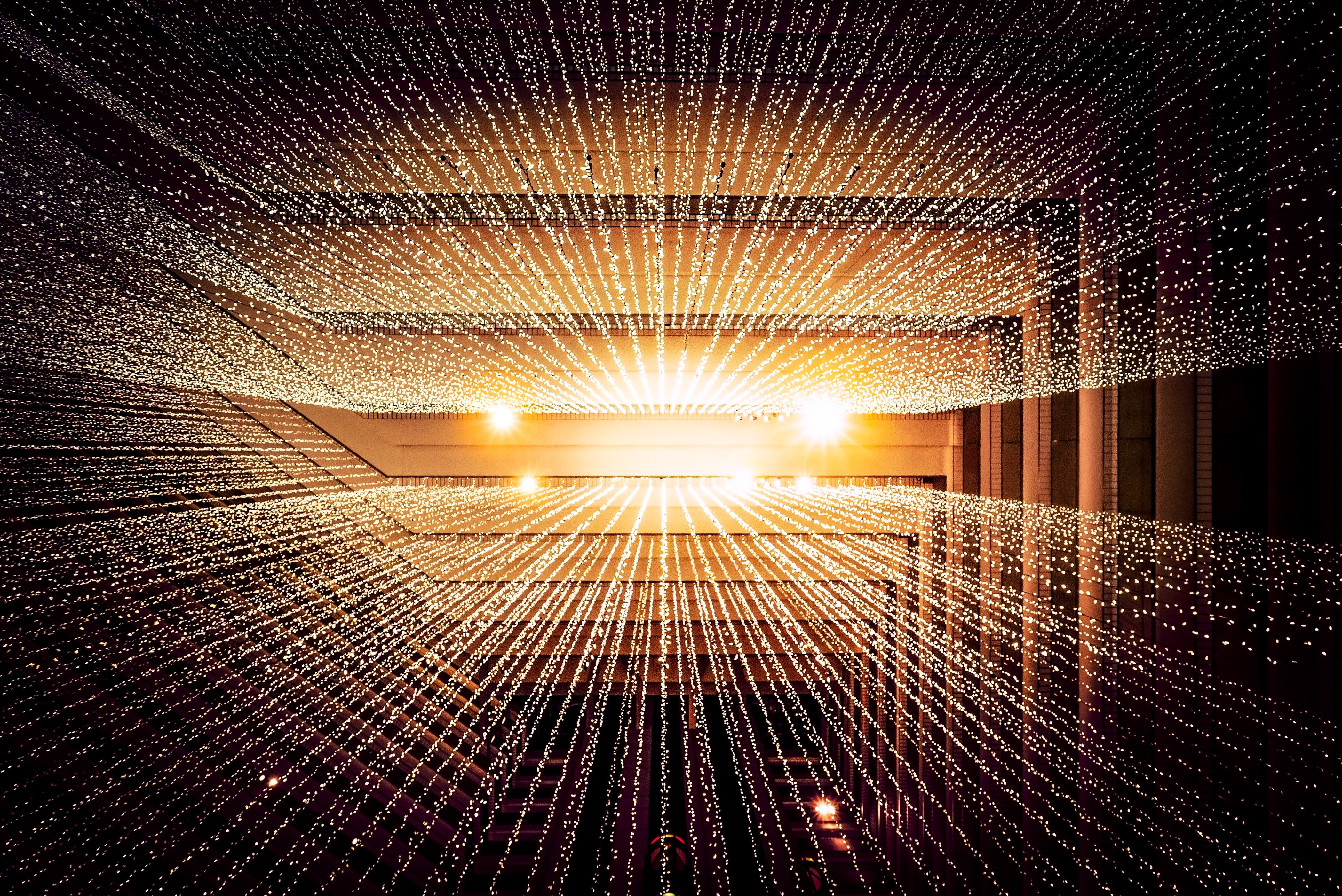
What is data governance and why does it matter?
Gartner defines data governance as “the specification of decision rights and an accountability framework to ensure the appropriate behavior in the valuation, creation, consumption and control of data and analytics.” Forrester says, “There is nothing simple or basic about data governance. Effective data governance grows out of data management maturity. It is why, to make progress, organizations are hiring chief data officers and activating strategic and unified data, analytics, and data governance competency centers.”
Data governance isn’t straightforward in the public sector. The demand for data and analytics often outpaces the development of governance. There are vast amounts of citizen data, a need for strict security and disparate data sources. This is made more difficult by the existence of data silos, different types of users and legacy implementations, all of which lead to multiple forms of governance split across government departments and functions. On top of that, there are emerging technologies that need to be continuously monitored, assessed and built into your data strategy.
5 benefits of data governance
Data governance realizes local value, provides quality data at scale and ensures data security. But it is not a quick-fix solution. You will need to adapt your framework to future technologies and business critical projects. But, in the long term, the strategic benefits outweigh the effort:
- Data security is defined and implemented to mitigate the impact of big data projects and the continued migration to cloud platforms that can create new threats
- Consistent view of data whilst enabling localized flexibility to reduce the duplication of efforts and provides clarity for decision-making
- Improved data quality with standards that ensure accuracy, completeness and consistency
- Single version of the truth that enables cross-enterprise transparency and compliance and data reuse
- Increased resilience to changes in technology, regulations, best practices and security by creating a consistent framework to monitor and assess them
How to ensure good data governance
Connect data governance with business outcomes
Gartner emphasizes the importance of “identifying the strategic business outcomes and relative priorities” that link to data governance. Governance frameworks can then be tested on specific use cases that have critical business implications. This creates a nuanced approach to implementing data governance, where you can monitor when it fails, why and how to adapt it to present and future use cases.
Create a collaborative governance approach
To be an agile data enterprise, you need to drive your governance decisions out of stakeholder collaboration and buy-in. Workshops with teams across functions can help to define the required business outcomes, data quality, assets and future data context and development. Then you can develop a risk-aware data governance plan that takes into account local and centralized needs and future changes in data technologies. After all, collaboration will reinforce best practices and create a culture of enablement and sustainability.
Remember that one size does not fit all
The complexity of technology means that different data governance styles are needed for different technologies. Gartner defines this as adaptive analytics and identifies four core styles for enterprise maturity. These are:
- Control: Based on enforced rules, policies, standards, and directives. This is best applied to Master Data Management (MDM) and regulatory compliance (i.e. GDPR).
- Outcomes: Focused on driving business outcomes whilst balancing risk and return on investment. This form of governance works with dynamic processes that change the way an enterprise works such as the multichannel citizen experience.
- Agility: Enables local teams to make decisions that create business value. This applies to ways of working and core competencies and supports self-service analytics and innovation labs.
- Autonomous: Drives value and manages risk from decisions made by people and technologies in real-time. So, it’s often used for autonomous decision-making in fraud detection or Machine Learning algorithms.
This new, adaptive, form of data governance puts a strong emphasis on the kind of technology being governed and acknowledges that different technologies require different styles of governance. So, the traditional out-of-the-box governance model is out, replaced by models that build in the complexity of your enterprise model. A Public Sector organization can manage this by investing in delivering the value of governance at scale.
Final thoughts
Better data governance is a long-term business initiative, not an IT issue. To create a flexible model, IT and business stakeholders need to pull together to agree the expectations for data quality and to connect governance with business outcomes. This is critical for a fragmented public sector that will be measured by how successful it is in managing the massive expansion of data in the next decade.