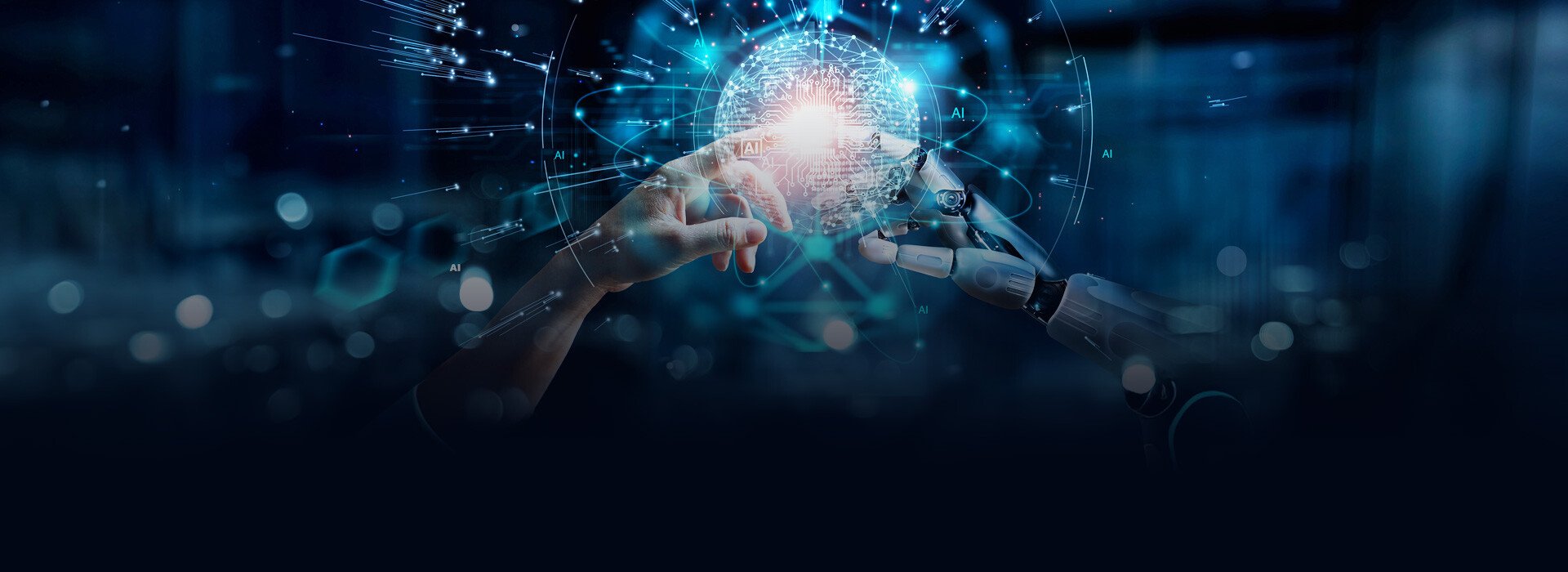
The transformative force of artificial intelligence (AI) and machine learning (ML) is an undeniable truth in today’s business landscape. Without a doubt, it has had a multiplier effect in redefining how organizations innovate, enhance efficiency, and unlock new revenue streams.
Even as a McKinsey report indicates that AI capabilities have doubled in the past five years, organizations find themselves challenged to scale their AI efforts. They encounter roadblocks of (a) longer delivery timelines, (b) difficulties in transforming proof-of-concept models to production-ready models, (c) risk of model obsolescence due to changes in underlying data or business requirements, and (d) challenges of security, regulatory, and ethical compliance requirements.
While these are real challenges, organizations must quickly find ways to effectively address and resolve them. The AI juggernaut has irretrievably moved into the fast lane and cannot slow down.
How can organizations leverage the power of advanced data analytics to scale AI and achieve winning outcomes?
Data quality — a paramount first
The most important requirement — and also the most challenging — for developing new AI and ML models is the quality of data. Data errors, missing values, bias, and outliers can adversely impact the development of AI models and the quality of their performance. If organizations have to generate meaningful, actionable insights from their data to build efficient AI models, there can be no compromise on data quality.
It is in this context that feature stores can play an important role in accelerating AI at scale. It is a centralized marketplace for storing, managing, and sharing features — on which AI/ML models depend to create consistency across different projects. A unified platform for data scientists to collaborate and reuse features can go a long way in removing duplication of effort and speeding up model development and deployment.
By continually enriching with new use cases, feature stores can enable data teams to reuse and repurpose them for their current and future use cases. This can significantly slash model development time and enable data teams to deliver outcomes of higher consistency. Version control of features can be maintained, data lineage can be tracked to boost the quality and governance of the AI/ML pipeline. Result? Higher accuracy of and trust in AI/ML models, better opportunities to nurture innovation and drive growth in today’s competitive environment.
Standards and protocols for immaculate data governance
Robust and uncompromising standards and protocols are absolutely important to scale up AI transformation with speed. They guide data teams to build, evaluate, and deploy the right AI/ML models with confidence. The right frameworks provide standardized approaches in developing AI use cases, with the right guardrails aligned to their respective industries.
Software engineering standards, data and ML lifecycle best practices, and compliance with regulations and ethics are three critical and non-negotiable aspects of data governance in scaling AI.
Standard software engineering technologies — such as continuous integration / continuous deployment (CI/CD), and automated testing frameworks — can maximize the value of AI investments. They establish a standard deployment pattern that can be integrated into the IT infrastructure. Additionally, they enable collaboration to reduce errors and enhance the quality of AI applications — thereby improving time-to-market.
The right data and ML best practices with clearly defined protocols can streamline the analytics process of data ingestion, engineering of ML features, and building and testing of models. It also allows for efficient performance monitoring after the model is deployed.
Most important is to ensure that uncompromising legal and ethical norms are set up to operate with the highest standards of responsibility from the very beginning.
A data-centric infrastructure to scale AI
An integrated environment of hardware (GPU, TPU, etc.) and software, specifically designed for AI/ML workloads is important for efficient data processing, model training, and deployment. This is strategically important to deliver flexibility and support in the scaling of AI through its lifecycle — and to ensure security and compliance.
While considering a data-centric AI infrastructure six aspects need to be kept in mind - data handling and storage, data processing, computational power, networking, security and compliance, and MLOps.
Also remember that AI infrastructure needs to be a dynamic ecosystem to facilitate optimized AI workflows of data gathering, pre-processing, model training, validation and deployment.
The concept of DevOps has revolutionized software development, and it's high time we applied similar principles to AI. This calls for establishing
- Robust MLOps pipeline for efficient model deployment and management
- Continuous integration and continuous delivery (CI/CD) pipeline for AI to streamline the transition from PoC to production
- Version controls for reproducibility
- Automated model training through cloud-based platforms
Analytics for frictionless AI
Robust analytics practices are critical to unlock AI’s transformative capabilities. They include the following:
- Data quality management through cleansing of inconsistencies, errors, and missing values
- Data preparation and feature engineering for training AI models
- Exploratory Data Analysis (EDA) to understand underlying trends, patterns, and relationships within data — and identify areas where AI solutions can deliver maximum impact.
- Data segmentation and targeting to train AI models for different business segments and processes
- Analytics tools to evaluate different AI model architectures and parameter settings for efficient resource allocation and maximized model performance
- A/B testing to compare different AI model versions
- Analytics techniques to enable explainability and interoperability
- Analytics dashboards for real-time monitoring and performance tracking
- Analytics tools to retrain AI models with new data or adjust parameters for optimal performance as business needs and data evolve
- Data visualization to translate complex insights into clear and compelling communication on AI’s impact to stakeholders across the organization
- Tools to measure the return on investment (ROI) of AI solutions in terms of increased revenue, improved efficiency, or reduced costs
Trust — a critical must for to scale AI
In our efforts to scale AI deployment, we must ensure that we have the trust of all stakeholders in the fairness, security and dependability of AI systems.
What does trust in AI actually mean? It is a multi-dimensional aspect that encompasses (a) transparency in decisions that AI models make, (b) how accountability is baked into the outcomes that AI is responsible for, and (c) how data privacy and ethical behavior are assured. Establishing trust calls for nurturing collaboration so that users feel that they have a say in the refinement of AI systems.
It should start right at the beginning when the AI vision and strategy are established. Communication plays an important role in fostering a culture of transparency and openness. Additionally, robust data governance frameworks, leveraging explainable AI technologies, and setting up rigorous testing and validation processes will add to the building of trust.
Data and analytics are the fuel that powers AI for both speed and scale — and key to staying ahead in today's dynamic business landscape. In doing so, one must remember that analytics and AI are deeply intertwined and complementary forces. To successfully achieve this end, organizations will need to
- Foster a data-centric mindset throughout the organization
- Build a skilled team of data scientists, machine learning engineers, and data engineers
- Build robust analytics practices through cross-functional collaboration between data, IT, and business — this will unlock the true potential of AI solutions and drive outstanding business impact
Relentless focus on data quality, data-centric AI infrastructure, talent-building and continuous improvement will give the surge needed to harness the transformative power of AI in a responsible manner. Above all, organizations need to embrace AI responsibly, with human-centric values and experiences.