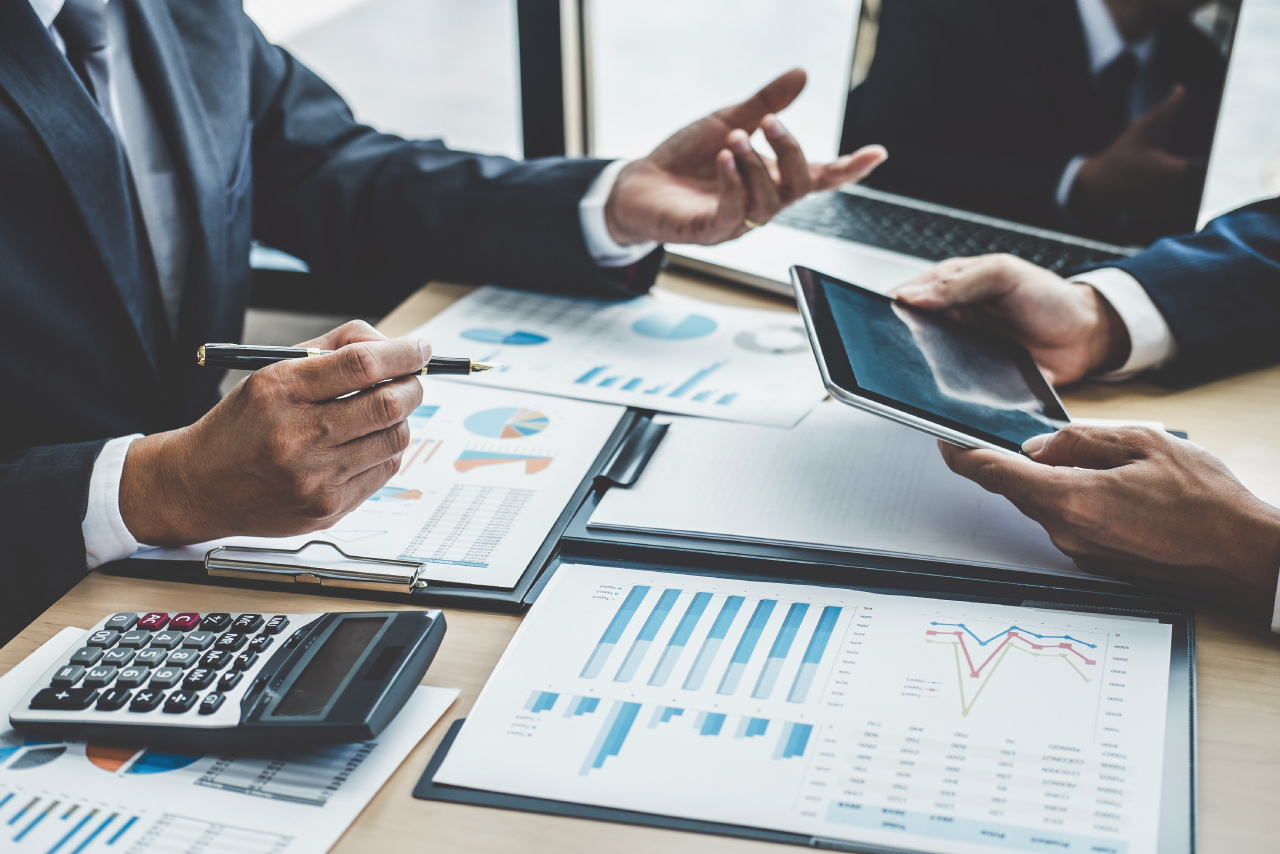
In today’s era, wherein smartphone banking is commonplace for most of us, it's easy to forget that billions still lack access to basic financial services - including the ability to save money safely, access credit for a small business, or protect themselves against financial emergencies remain, across both developing and developed economies. But the AI-led technological revolution could change everything.
As an AI enthusiast myself, with years of experience in the Finance domain, this is how I think technology can reshape the future of global financial access and what it takes to get it right.
The Promise of AI in Financial Inclusion
The COVID-19 pandemic unexpectedly accelerated financial inclusion worldwide. According to the Global Findex Database 2021, 76% of adults globally now have an account, up from 68% in 2017. Mobile money accounts have become particularly important in developing economies, doubling adoption rates since 2017.
Yet, significant challenges remain. Women, the poor, and those without formal education continue to have lower access rates. This is where AI enters the picture – not as a magic bullet but as a thoughtfully deployed tool with enormous potential.
Real Challenges and How to Address Them
Implementing AI for financial inclusion isn't without hurdles:
1. Limited Historical Data
Many potential customers have thin or non-existent formal financial histories. The solution? Alternative data sources and innovative modeling approaches.
2. Ensuring Data Quality
Data from developing markets may have inconsistencies or gaps. Robust data validation processes and local context expertise are essential.
3. Addressing AI Bias
Models trained on historical data may perpetuate existing biases. Diverse teams, explicit fairness metrics, and ongoing monitoring help mitigate this risk.
4. Balancing Innovation and Regulation
Financial regulations vary widely across markets and may not yet fully address AI applications. Close engagement with regulators and a commitment to responsible innovation are key.
Strategies That Work
1. Building the Right Team
Developing AI solutions for underbanked populations isn't just a technical challenge – it's a human one. The most successful implementations require diverse teams with complementary skills:
- Technical experts (data scientists, ML engineers, and GenAI specialists) providing the backbone of AI development
- Domain experts in microfinance and financial inclusion ensuring solutions address real needs
- Ethicists and legal experts to keep everything responsible and compliant
This multidisciplinary approach helps create holistic solutions that are technically sound, culturally appropriate, and ethically responsible. For example, when building a credit scoring model for informal sector workers, data scientists might incorporate alternative data sources while domain experts ensure the model recognizes unique cash flow patterns of street vendors or seasonal workers.
The challenge? Communication across disciplines isn't always easy. Organizations also need "translators" who can bridge technical, business, and ethical considerations. Another challenge is the need to cultivate continuous learning as both AI technology and microfinance evolve rapidly.
2. Choosing the Right Technologies
When it comes to technology choices for serving underbanked populations, there's no one-size-fits-all approach. Organizations must make thoughtful decisions about:
Cloud vs. On-Premises
Cloud solutions offer scalability and advanced AI services, but on-premise might be necessary for data localization requirements or areas with unreliable internet. Most successful implementations use a hybrid approach.
Open-Source vs. Proprietary
Open-source frameworks provide cost-effectiveness and flexibility, while proprietary solutions offer dedicated support and user-friendly interfaces. Again, a balanced mix often works best.
Mobile-First Approach
This isn't optional – it's essential. In many developing regions, mobile phones are the primary (or only) digital device. Solutions must work on basic smartphones with intermittent connectivity. Think lightweight apps, progressive web applications that function offline, and even SMS-based services.
I've seen firsthand how these technological choices directly impact reach and accessibility. A cloud-based solution might work brilliantly in urban centers but fail completely in rural areas with spotty connectivity. Mobile-first design isn't just good practice – it's the difference between reaching your audience or missing them entirely.
3. Phased Implementation
As a finance expert at Mastek, I suggest that when deploying AI for financial inclusion, rushing to scale is tempting but risky. A measured approach works best:
a. Pilot programs in select markets could help test solutions in real-world conditions while minimizing risks.
b. Gradual scaling with continuous monitoring will help identify issues before they affect large populations.
c. Regular stakeholder feedback from customers, employees, regulators, and community organizations helps shape ongoing improvements.
This approach balances caution with progress. For example, after successfully piloting an AI-driven loan application process in one region, you might discover through customer feedback that the interface confuses some users. Addressing this before wide deployment saves resources and builds trust.
4. Measuring KPIs with Purpose
How do we know if AI is truly driving financial inclusion? It requires tracking both business metrics and social impact:
Business Performance Indicators
- Increase in new accounts from underserved populations
- Reduction in loan default rates
- Improvement in customer satisfaction scores
- Time-to-decision for loan applications
- Operational efficiency gains
Social Impact Metrics
- Increase in savings rates among previously unbanked people
- Growth in small business loans in underserved areas
- Improvement in financial literacy scores
- Digital financial service adoption rates
- Women's economic empowerment indicators
- Community economic resilience
What's particularly powerful is connecting these metrics. For instance, when improved AI credit scoring leads to more accurate risk assessment, it enables lending to previously excluded borrowers while maintaining healthy default rates. According to PwC analysis, lenders are already seeing 15-30% increases in credit approvals with no change in loss rates – expanding access while maintaining sustainability.
The Path Forward is Based on Responsible Innovation
The Global Findex Database 2021 shows promising progress, but there's still work to do. Nearly a quarter of adults worldwide remain unbanked, with persistent gaps along gender and income lines. AI offers powerful tools to address these disparities, but only if deployed thoughtfully.
The organizations making the greatest impact share common traits:
- They maintain a laser focus on customer needs
- They invest in diverse teams and local expertise
- They choose technologies appropriate for their context
- They implement solutions gradually with continuous learning
- They measure both financial and social outcomes
Financial inclusion isn't just about account numbers – it's about creating pathways to economic opportunity and resilience. When AI solutions are designed with this broader purpose in mind, they become powerful catalysts for positive change.
As we look ahead, the most exciting innovations will likely come from collaborations between financial institutions, fintech startups, technology-led companies, and community organizations. By combining technological capabilities with deep local knowledge, these partnerships can develop AI solutions that are not just technically impressive but genuinely transformative for underserved communities.
The journey toward AI-driven financial inclusion is complex, but the destination – a more equitable financial system that works for everyone – is well worth the effort.